Organizations often prioritize productivity and efficiency, relying heavily on metrics and performance indicators. However, this focus on quantitative measures can sometimes obscure the broader context, leading to unintended consequences and skewed results. This article examines the nuances of measuring productivity, the dangers of relying too heavily on specific metrics, and the need for a comprehensive approach to performance evaluation.
Beyond the Metrics
In the ever-expanding realm of data, information can resemble a dense and complex jungle. Countless numbers lie before us like a vast, unexplored wilderness. While seemingly endless metrics surround us, the ability to critically analyze them remains paramount. This article explores the core principles of effective data analysis, emphasizing th…
In my previous article, I offered insights from observing how organizations deal with adversity. To follow the structure of the OODA Loop, it is now time to focus our strategies on building resilience and move to the next stage: Orient. It means building systems that don't just bounce back, but evolve. For example, if hiring metrics stall under pressure, it may be necessary to reevaluate current practices. Are they too rigid? Do we need more adaptive, robust approaches? While observing chaos can lead to despair, it's important to remember that an abundance of data doesn't always equate to greater understanding. The key is thoughtful analysis and interpretation of information to drive meaningful change and build organizational resilience.
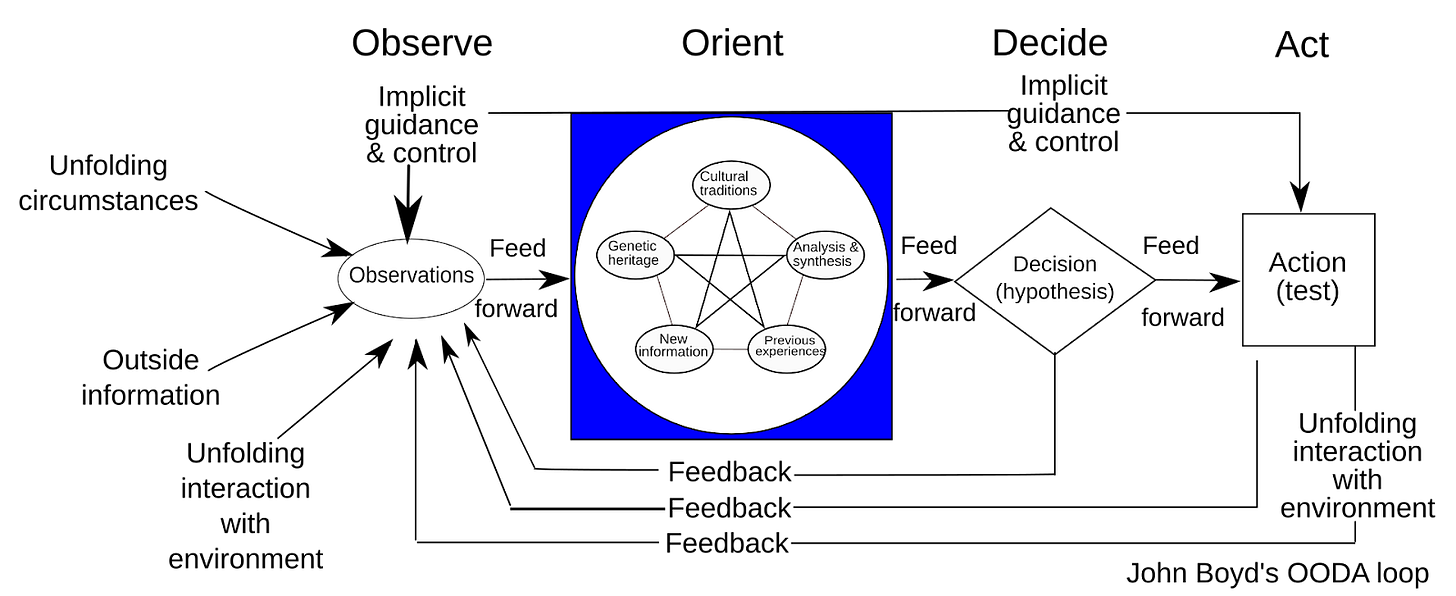
Mind over matter
Our society's fixation on productivity often values speed over substance, echoing Frederick Taylor's industrial-era methods. While Taylorism revolutionized production, its application in today's knowledge-driven landscape can be counterproductive. This approach, emphasizing rapid output and repetitive tasks, often leads to burnout and diminished work quality. Industries prioritizing quick turnarounds at the expense of thoughtful solutions exemplify these pitfalls. Simply put, knowledge workers are not factory workers.
David Kadavy's "Mind Management, Not Time Management" highlights how this speed-centric approach can backfire in modern work environments. It neglects the crucial elements of thoughtful problem-solving and strategic thinking, which are essential for sustainable productivity. Modern thought leaders like Naval Ravikant advocate for "earning with your mind, not your time"1, emphasizing the importance of quality thinking over mere speed. There is a difference between "Did I solve the problem quickly?" and "Did I solve the problem from recurring?”
Perfection in productivity is an illusion; unexpected events are inevitable. Operating at 100% capacity sets the stage for failure by eliminating flexibility. The authors of "Agile HR"2 suggest aiming for 70% capacity, allowing room for holidays, sickness, emergencies, and unplanned work without overwhelming the system. Companies adopting this practice often maintain steady productivity levels while avoiding burnout and high employee turnover. Maxing out processing power leaves no room for innovation. You're always catching up on what you've missed.
A successful organization requires a shift in focus from isolated metrics to a systems thinking approach, because organizations have a web of interactions and feedback loops. Systems thinking considers the interconnectedness of departments and roles within an organization. Russell Ackoff aptly observed: "Managers are not confronted with problems that are independent of each other, but with dynamic situations that consist of complex systems of changing problems that interact with each other. I call such situations messes. Managers do not solve problems, they manage messes."3
Worst day scenario
In teams that are constantly working at full capacity, prioritization may seem pointless. But crisis management shows why these systems are critical. Backlogs that are manageable in calm times can become overwhelming in a crisis. Without clear Service Level Agreements and prioritization, valuable energy is wasted on reactive firefighting. Our focus on customer satisfaction often leads us to view unmet SLAs as indicators of operational problems. This perspective requires a granular understanding. The answer is most likely to be found in the data, using process or task mining.
Constantly overworked teams lack the mental space to proactively solve problems. For example, during a major system outage, teams with well-defined SLAs can prioritize critical fixes over less urgent tasks, ensuring efficient resolution of critical issues. During my company's recent ServiceNow implementation, we faced a critical question: Do SLAs and prioritization systems remain relevant under extreme pressure? The two-week work stoppage created a massive backlog of work for the new system (with some requests duplicated or tripled), resulting in significant delays, frustration, and confusion.
Some managers avoid detailed data analysis, believing they can handle crises by talking to their team. They often prefer plausible narratives to explain temporary problems rather than understanding the root causes of team overload and preventing its recurrence. This avoidance typically stems from a lack of trust within the organization or a lack of data analysis skills. However, in-depth analysis can reveal systemic issues that, once addressed, can lead to more sustainable operations. Managers should embrace data-driven decision making and use tools and training to improve their analytical skills. Understanding team performance during challenging times provides a realistic picture of resilience and adaptability. Sure, you know when your team is overworked, but maybe you'd like to know why.
Shifting the focus from "best day" to "worst day" performance allows for deeper analysis of systems and processes during challenging moments. For example, analyzing customer service response times during peak complaint periods can identify process bottlenecks and areas for improvement that aren't apparent during normal operations. Identifying weaknesses exposed under pressure builds resilience and promotes continuous improvement, essential for long-term success. As the saying goes, "we don't rise to the level of our expectations; we fall to the level of our training."
Our worst days expose the gaps in our preparation and highlight areas for growth. While talent and potential matter, the ability to execute consistently, even on uninspired days, distinguishes truly successful individuals and teams. By examining our response to challenges and setbacks, we can develop targeted strategies to improve our resilience. This may include implementing training programs that simulate crisis conditions or establishing regular review processes to learn from past incidents. Such analysis provides valuable insights into ourselves, our teams, and methods for building resilience for the inevitable difficult times.
Diamonds are shaped under pressure
I once worked in a highly dynamic startup environment where plans changed quickly and pivots were the norm. Some people thrive in this environment, appreciating the flexibility and innovation, while others prefer more structure. I enjoyed the adaptability and open-mindedness, but I also noticed a lack of accountability when quick decisions were made. It's important to learn from the feedback on our decisions once they are implemented. Without experiencing the results, it's hard to understand what went wrong and how to improve. Long-term side effects can occur months after the decision is made.
Changing KPIs from month to month before adjusting the ways of working is like fixing bugs in production: you unknowingly create new dependency problems. Without allowing time for adjustments to take effect and generate meaningful feedback, it's impossible to understand the true impact of the changes. Consistency in data collection over time (even with some internal, but consistent errors) may reveal the unexpected trend. This is a better approach than trying to overfit the data to meet expectations of the management.
Measuring team performance, particularly during high-stress periods, requires looking beyond surface-level metrics. For example, a spike in high-priority backlog items during a large project reveals problems with prioritization and resource allocation. Monitoring SLAs Met is also crucial; a significant drop during pressure points indicates a need to improve processes or increase capacity. Similarly, an increase in first response time during busy periods may suggest team overwork or inefficient workflows.
While quantitative metrics provide a clear performance picture, qualitative data offers insights into the team's experience. Employee sentiment surveys provide valuable information about stress levels and the ability to handle pressure. Understanding how your team feels during challenging times helps identify areas needing support or adjustment.
The larger context is also important. Tracking metrics such as customer satisfaction and time to resolution, especially during high-pressure periods, would help. They would show whether the team is maintaining quality service when stretched thin. In addition, monitor the number of escalations; an increase may indicate a need for additional training or improved communication. Siloed knowledge can easily lead to parallel processes where no one is on the same page anymore.
Combining these quantitative and qualitative measures gives managers a comprehensive view of team performance under pressure. It helps make informed decisions about prioritizing tasks, allocating resources, and building overall team resilience. As Daniel Kahneman argues, the more noise there is, the more the company should care about process, not outcome, if workers can't do much about it.4 A solid process helps eliminate distractions and maintain focus, ensuring that the team can effectively overcome challenges and consistently improve performance.
Interconnected system
A systems-thinking approach to recruiting is essential. Recruitment interacts with multiple factors, such as employer branding, the quality of the talent pool, and the onboarding experience. For example, a high interview rejection rate may not only reflect the quality of candidates, but may also indicate unclear job descriptions or an unrefined interview process. Analyzing these aspects holistically provides a more accurate picture.
Consider a scenario where a company has a high offer acceptance rate (OAR), but experiences a significant drop-off during onboarding. This could indicate a disconnect between the job description and the actual role, highlighting the need to consider employer branding and a smooth onboarding experience alongside core recruitment metrics.
Analyzing attrition data can yield surprising results. In "Agile HR," Natal Dank and Riina Hellström describe a case where an HR team considered redesigning its graduate program after noticing a high dropout rate among new hires before their start date. Initially, the data suggested the need for significant changes. However, deeper user research and prototyping revealed a simpler solution: a welcoming phone call from the new manager to the graduate. This small gesture significantly increased retention by making graduates feel valued and connected. The case highlights the importance of addressing broader HR issues and the value of user-centered solutions.
While Time to Fill and Time to Close are important recruiting metrics, they measure different aspects of the hiring process. Time to Fill measures the time from job posting to acceptance but only considers successful hires, potentially inflating recruiting performance. Time to Close provides a more accurate measure by including all requisitions, capturing the full scope of the recruiting process and offering a comprehensive view of invested time and resources.
Remember that individual tool usage doesn't always affect the overall outcome. Consider external factors like interview scheduling delays, feedback timeliness, offer negotiation, and competitive offer development. Investigate each case further, evaluating message quality, profile content, search effectiveness, and job requirement complexity. Examining these areas helps HR identify and address inefficiencies, improving the hiring process and aligning with strategic goals.
Many HR inefficiencies stem from a siloed operating model where different teams manage recruiting and talent development separately. This division, which often mirrors the pillars of the Ulrich model, leads to process bottlenecks such as delayed hiring team decisions or overly burdensome assessments. These inefficiencies result in candidate attrition, longer time to hire, compromised recruiter capacity, and increased costs. The more people who have to make decisions, the longer it takes, especially in countries with a consensus-driven culture like Germany.
Mismanagement
The Peter Principle suggests that people often rise to the level of their incompetence. Many organizations, due to growth, attrition, or hiring challenges, promote individuals to management positions without the proper training, skills, or desire. This is often the only path to career advancement, even for highly specialized individuals. Not all organizations offer parallel Individual Contributor roles for career growth, leading to frustrated managers without a true calling or individuals seeking opportunities elsewhere. While managers can learn on the job, some choose this role out of necessity rather than genuine interest.
Managing team members and their workloads requires different skills. Data analysis can provide clarity, but managers' comfort level with numbers varies. Some are data savvy, while others prefer direct guidance. As Selena Fisk notes in "I am not a numbers person": "Beyond the scepticism throughout the pandemic, there are, more generally, large numbers of our population who fear data and numbers. For many, this stems all the way back to their experience with maths in school, particularly as they moved into secondary school (Dowker et al., 2016). Many refer to this phenomenon as 'maths anxiety' – and it's not a new phenomenon."5
Simply repeating "we are data-driven" can be a misleading mantra if actions do not support the slogan. Instead, the focus should be on developing data literacy, because being blindly driven by data can lead you astray. While statistics don't lie, human interpretation can be flawed. Some people interpret data outliers as significant indicators without considering the broader context. The aforementioned author warns against this trap, stating: "The reality is that your revenues, expenses, profits, and sales will fluctuate - they will not consistently increase, decrease, or stay the same. That's why one point higher or lower than the last is not a trend - wait for a third piece of data before you even think about making statements about trends rather than each day's raw data."6 For some insights, excluding the top 5 or 10 percentile can improve statistical significance and prevent skewed data.
But data alone doesn't paint the whole picture. The human element remains critical. Team dynamics, training, and potential biases in data interpretation all impact the hiring process. For example, a team with strong collaboration skills can leverage diverse perspectives to make better hiring decisions. Investing in unconscious bias training can mitigate its impact on candidate selection.
Daniel Kahneman observed in "Noise: A Flaw in Human Judgment," that people often form quick impressions when meeting someone new. First impressions during interviews often focus on superficial attributes such as charisma and verbal skills. Even the quality of the handshake can significantly influence hiring decisions. This demonstrates our tendency to look for patterns and construct narratives from minimal information. In one experiment, researchers found that interviewers felt they learned as much about candidates who gave random answers as those who gave genuine answers, without realizing that the answers were randomon purpose. This highlights our tendency to prioritize immediate impressions and construct meaningful narratives from limited information.
Work more to get more
Managers' expectations significantly influence others' behavior. Self-fulfilling prophecy experiments have shown that students tend to live up to the expectations of their teachers. This phenomenon is often referred to as the "Pygmalion Effect," and it is no different in the workplace. It's critical to protect individuals from biased expectations during both the hiring and evaluation processes. Unfortunately, this is a lot easier said than done.
The distribution of work is paradoxical: high performers often get more work, while talented employees may be overlooked if they can't showcase their skills. Those who excel at certain tasks, such as reporting, often end up with more of the same, depriving others of learning opportunities and themselves of growth in new areas. This creates a cycle known as the Matthew Effect, where "the rich get richer," a term coined by sociologist Robert Merton.
To break this cycle and build a well-rounded team, encourage skill diversification by assigning tasks outside people's comfort zones. This approach helps develop generalists, increases internal mobility, boosts motivation, and improves retention. Embrace the concept of "giving your Legos to others" - sharing tasks to help team members develop new skills and avoid knowledge silos. By distributing tasks and responsibilities, you create an environment where all team members can develop new skills and contribute more broadly to the organization's success.
Managers should actively rotate responsibilities, provide training opportunities, and encourage knowledge sharing to create a culture where people feel safe to take on new challenges without fear of failure. Regularly reviewing and adjusting job assignments ensures that everyone has the opportunity to develop a broad skill set, promoting a dynamic and adaptable team. Staying in narrowly defined niche roles, while sometimes prestigious, can lead to stagnation and limited career growth. Moreover, as recent news stories have shown, those confined to such roles may be at greater risk of being replaced by automation and AI technologies.
In the context of AI implementation, a new paradox emerges: Language Learning Models (LLMs) can level skills between junior and senior staff, or even between technologically advanced and developing countries. Subject matter expert Ethan Mollick, who coined the term "co-intelligence" (in his eponymous book) as an optimal way to work with AI, noted: "Just because early results for AI suggest that only lower-performing people benefit does not mean that this is the only possible pattern. It may be that the reason only lower performers gain from AI currently is that the current AI systems are not good enough to help top performers."7 While ChatGPT may initially benefit lower-level performers, more advanced AI systems are likely to provide greater benefits to top performers. These individuals can initiate ambitious projects and use AI to execute them effectively. The long-term impact on the skills gap remains uncertain. As these tools evolve, adaptability will be critical to their effective use. Those who have long secured their roles and do not actively seek to move out of their comfort zones often lack this adaptability.
Better be lucky than good?
Evaluating specific skills can sometimes lead to mistaking luck for real effort. For example, evaluating a tech sourcer's performance based solely on raw numbers can be misleading. In my own experience, I have observed that some sourcers can consistently land superstar hires. However, this begs the question: is their success due to pure skill, or is it simply unsustainable luck? A short-term performance sample can't capture true ability due to the "law of small numbers," where tasks must be continuously repeated to reveal true ability. When faced with obstacles, would these sourcers find original solutions or rely on early career tactics?
Daniel Kahneman introduced the concept of regression to the mean, which we often overlook by focusing too much on outliers. When evaluating performance, it's important to distinguish between skill and luck, while recognizing regression to the mean. Recruitment results fluctuate - what initially appears to be luck may be skill, and vice versa. Over time, performance stabilizes around a true mean. Shane Parrish has aptly described this challenge: "Talent and potential mean nothing if you can't consistently do the boring things when you don't feel like doing them."8
Often, performance outliers are the result of natural variance rather than true ability or effort. Understanding the goals and processes of different activities is essential. For example, the strategies for sourcing a software developer versus a sales executive are very different due to the nature of the roles and the skills required. The approaches used by a sourcer - whether mapping, pipelining, contacting, or reviewing - require tailored metrics. Each style, from the systematic searcher to the opportunistic hunter, impacts results differently. These nuances aren't immediately apparent from raw data alone. Understanding these dynamics allows for more accurate performance assessment, ensuring fair and targeted reviews.
Analyzing performance requires understanding the relationship between effort and outcome, often thought of as a balance between luck (lottery) and skill (math problem). The included graphs illustrate this concept with two scenarios. In the first, a significant difference (Cohen's d = 2) between low and high effort indicates that effort has a large effect on outcome. In the second, a smaller difference (Cohen's d = 0.5) suggests that outcomes depend more on luck than effort. Knowing where your environment falls on this spectrum can help you set realistic expectations and strategies.
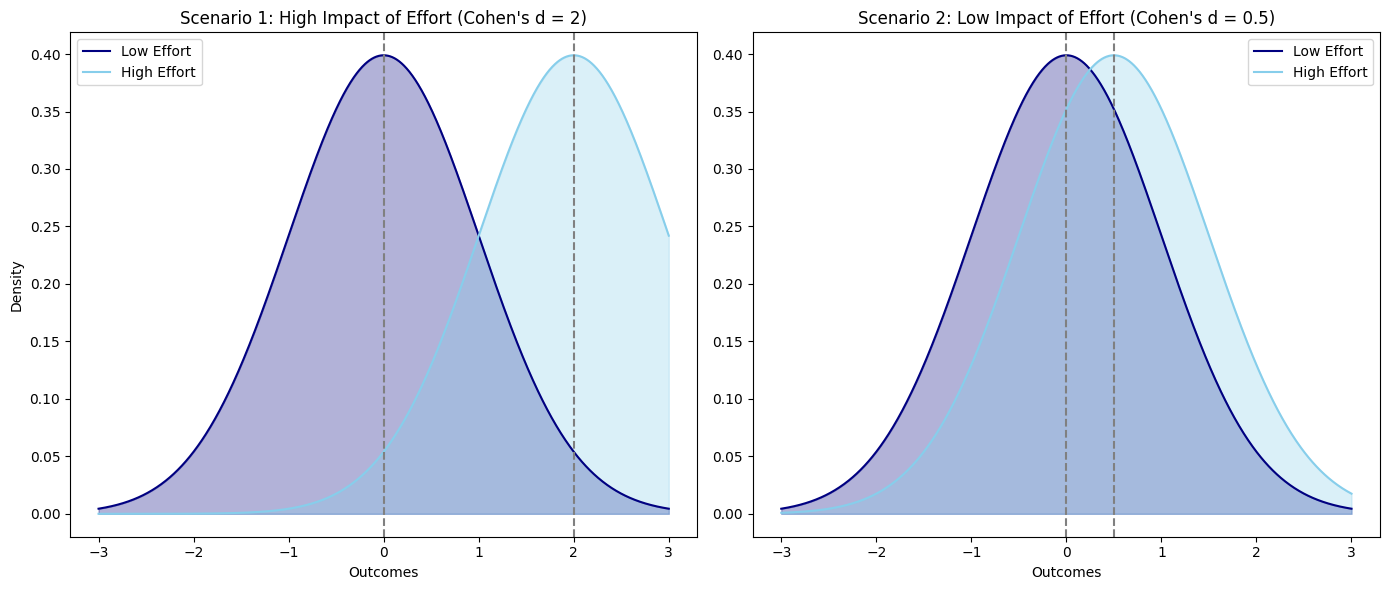
The professors of the People Analytics course at Wharton University9 suggest asking the following questions:
Are the differences persistent or random? I.e., how do we know this isn't just good/bad luck?
Is the sample large enough to draw strong conclusions? How can we make it larger?
How many different signals are really tapping into here? How can we make them as independent as possible?
What else do we care about? Are we measuring enough? What can we measure that's more fundamental?
Applying these insights into recruiting and HR analytics means assessing both individual performance and context. By considering situational factors in addition to personal attributes, organizations can create a fairer, more comprehensive assessment framework. This approach aligns with principles such as Central Limit Theorem, which stress the importance of large sample sizes for reliable data. To reduce the influence of chance, focus on skill-related performance measures, which persist over time, while chance-related measures regress to the mean.
Gaming the system
Metrics in performance management are a double-edged sword. Used poorly, they can create a toxic environment of public shaming, demotivation, and misleading tactics to achieve goals. This narrow focus on metrics can overshadow broader goals, causing employees to neglect important aspects of their jobs. Metrics are tools, not goals. Overemphasizing them can distort the original intent and harm the organization.
American social scientist Elton Mayo studied the effects of lighting on worker productivity in his Hawthorne experiments. Surprisingly, productivity increased by 25% regardless of changes in physical conditions. This phenomenon, now known as the Hawthorne Effect, occurred because workers felt valued and empowered by participating in the experiments. Mayo's findings shifted the focus from Taylorism, which emphasized speed and efficiency, to the human relations movement, which posited that meeting workers' social needs significantly increased productivity.
Erik Van Vulpen warns in "The Basic Principles of People Analytics"10 that any change in the workplace, especially if employees know they're being watched, can temporarily boost performance. A similar concept was highly publicized years ago when Volkswagen manipulated emissions tests to show better performance, but only when under scrutiny. Therefore, understanding context and potential bias is critical when interpreting performance metrics. This means that when setting KPIs and OKRs, managers must ensure that they truly reflect the team's work environment and aren't skewed by temporary improvements due to increased monitoring. Involving team members in KPI discussions and aligning them with organizational goals helps create meaningful and sustainable performance metrics.
When the focus is on the metric instead of the goal, it becomes problematic. Alan Benson's paper, "Do Agents Game Their Agents' Behavior?"11 examines how sales managers manipulate staffing and incentives to meet quotas. The study finds that managers often retain poor performers and adjust quotas to meet personal goals, especially when quotas are close to being met. While this behavior benefits managers in the short run, it can be costly to firms by retaining ineffective employees. Even without detailed reports, colleagues on the same team are subconsciously aware of how much everyone else is doing, which can lead them to question: "Why should I care more if they don't?" And that becomes a long-term morale problem for the entire organization, resulting in a loss of efficiency.
To avoid these pitfalls, take a balanced approach. Ensure that metrics are aligned with your goals, and use them alongside qualitative factors in a positive and collaborative work environment. That way, metrics become a compass that guides improvement, not a hindrance. You don't have to worry about people gaming the system if the incentives are right, and you can rely on their character traits. As Mark Manson said, “Competence is how good you are when there is something to gain. Character is how good you are when you have nothing to gain. People will reward you for your competence, but they will only love you for your character.”12
Measure is not a goal
Goodhart's Law, a principle in economics and statistics, states that when a measure becomes a goal, it ceases to be a good measure. This concept, named after British economist Charles Goodhart, was formulated in the context of monetary policy, but has found broad application in a variety of fields, including management, education, and public policy.
When a particular metric becomes the sole focus, it can lead to incentive distortion, where individuals or organizations optimize for that metric at the expense of other important factors or the overall goal. In addition, the behavior or system being measured may change, making the metric less reliable or representative of the original phenomenon. To illustrate, consider the education system. If schools are judged solely on test scores, teachers may be forced to "teach to the test" rather than focus on providing a holistic education.
Consider a help desk where success is measured solely by the number of tickets closed, which incentivizes agents to prioritize easy wins and leave complex issues unresolved. This "gamification" of support backfires. Easy tickets get resolved quickly, while difficult tickets pile up. Agents working on difficult issues become overwhelmed, and average resolution time suffers. Initial efficiency gains disappear as the backlog grows. Closing tickets is important, but it shouldn't come at the expense of quality or service. A more holistic approach that considers factors such as complexity and resolution time would incentivize agents to handle the right tickets, not just the easy ones.
The most complex example comes from the area of measuring the performance of software developers. This is inherently challenging due to the multifaceted nature of their work and can lead to a cascade of unintended consequences, illustrating the Cobra Effect. If developers are incentivized to write more code, they may add redundant lines and unnecessary comments to inflate their output. Conversely, if developers are incentivized to write shorter code, they may remove necessary comments or write overly concise code that is difficult for others to understand or maintain. A developer working on a complex algorithm may produce less visible output than a developer fixing simple bugs, but the former's contribution may be far more critical to the project's success.
To mitigate the effects of Goodhart's Law, organizations and policymakers must adopt a more nuanced approach to measurement and goal setting. This includes regularly reviewing and adjusting metrics, adopting a balanced scorecard approach that uses multiple indicators, and maintaining a holistic perspective on overall goals and objectives. In this way, they can ensure that metrics guide improvement without distorting the original intent.
Studies by Gollwitzer in 1997 and earlier13 show that the use of implementation intentions can increase the likelihood of successful goal attainment. This approach involves predetermining a specific, desired behavior in response to a particular future event or cue. Setting the right implementation intention can influence the right outcomes. The selected goal-directed behavior (the then-part of the plan) is performed automatically and efficiently, without conscious effort. Automating behavior in response to a future situation or cue eliminates hesitation and deliberation when a critical situation arises. This frees cognitive resources for other tasks and helps avoid goal-threatening distractions or competing goals. Once set, an implementation intention operates unconsciously, a process known as strategic automaticity.
Final words
The road from raw data to actionable insights is filled with potential pitfalls, but it's a necessary one for organizations that want to thrive in our rapidly evolving workplace landscape. As we've seen, metrics are powerful tools, but they must be used with care and context. The true art lies not in perfecting a single metric, but in orchestrating a symphony of indicators that together paint a comprehensive picture of performance and progress.
Looking ahead, as AI and automation are reshaping the nature of work, our ability to responsibly interpret and apply data will become even more critical. But in the midst of this technological revolution, we shouldn't lose sight of the human element. The most effective leaders will be those who can bridge the precision of data with the nuance of human judgment, creating an environment where both metrics and people can thrive.
This reminds me of a great quote from Albert Einstein: "The mere formulation of a problem is far more often essential than its solution, which may be merely a matter of mathematical or experimental skill. To raise new questions, new possibilities, to regard old problems from a new angle requires creative imagination and marks real advances in science."14
Ultimately, our goal should not be to achieve perfect measurement, but to foster a culture of continuous learning and adaptation. By embracing the complexity we've discussed and treating our metrics as guides rather than dogma, we can build more resilient, innovative, and ultimately successful organizations. You have to learn not just how to measure, but what really matters.
-- Michael Talarek
This is part 2 of a larger article consisting of 4 parts. More to come soon. Previous article is linked here:
Decisive Actions in the Data Jungle
In my last two articles, we delved into how to Observe and Orient within the data landscape. Now, let's move on to the next phase of the OODA Loop: Decide. Now, let’s focus on the next step in the OODA Loop: Decide. A year ago, when my company restructured the setup with new tools, I was thrust into a new team by my former manager. The challenge was to …
David Kadavy, “Mind Management, Not Time Management”
Natal Dank and Riina Hellström, “Agile HR: Deliver Value in a Changing World of Work”
Donella Meadows, “Thinking in Systems: A Primer”
Daniel Kahneman, “Noise: A Flaw in Human Judgment”
Selena Fisk, “I'm not a numbers person: How to make good decisions in a data-rich world”
Selena Fisk, “I'm not a numbers person: How to make good decisions in a data-rich world”
Ethan Mollick, “Everyone is above average” | https://www.oneusefulthing.org/p/everyone-is-above-average
Shane Parrish, “Adult Life” | https://fs.blog/brain-food/october-15-2023/
People Analytics course at Wharton University (Coursera) | https://www.coursera.org/learn/wharton-people-analytics
Eric Van Vulpen, “The Basic principles of People Analytics” | https://www.aihr.com/resources/The_Basic_principles_of_People_Analytics.pdf
Alan Benson, “Do Agents Game Their Agents' Behavior? Evidence from Sales Managers” | https://papers.ssrn.com/sol3/papers.cfm?abstract_id=2235922
Mark Manson, How to Develop Character | https://markmanson.net/breakthrough/045-how-to-develop-character
Implementation intention | https://en.wikipedia.org/wiki/Implementation_intention
Leopold Infeld, Albert Einstein, “The Evolution of Physics: The Growth of Ideas from Early Concepts to Relativity and Quanta”